Predictive Maintenance in Smart Factories: Maximizing Productivity and Minimizing Downtime

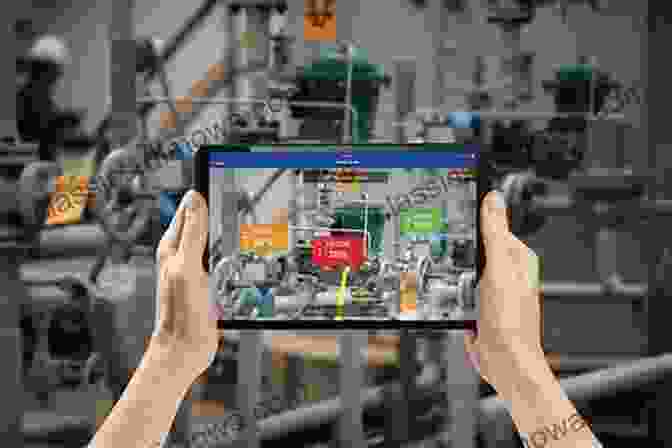
In the стремительно развивающийся era of Industry 4.0, where digitalization and automation are transforming manufacturing processes, predictive maintenance (PdM) has emerged as a game-changer for smart factories. By leveraging advanced data analytics and machine learning (ML) techniques, PdM empowers manufacturers to proactively identify and address potential equipment failures, ensuring optimal uptime and maximizing productivity.
What is Predictive Maintenance?
Predictive maintenance is a proactive maintenance strategy that utilizes real-time data from sensors, devices, and machines to predict when equipment is likely to fail. Unlike traditional reactive maintenance, which involves repairing or replacing equipment after it has failed, PdM allows manufacturers to take preemptive action, preventing costly breakdowns and reducing downtime.
4 out of 5
Language | : | English |
File size | : | 35138 KB |
Text-to-Speech | : | Enabled |
Enhanced typesetting | : | Enabled |
Word Wise | : | Enabled |
Print length | : | 401 pages |
Screen Reader | : | Supported |
Hardcover | : | 430 pages |
Item Weight | : | 1.43 pounds |
Dimensions | : | 6.14 x 0.94 x 9.21 inches |
X-Ray for textbooks | : | Enabled |
Benefits of Predictive Maintenance in Smart Factories
Implementing predictive maintenance in smart factories offers numerous benefits, including:
* Increased uptime: By proactively addressing potential equipment failures, manufacturers can avoid unplanned downtime and maintain continuous production. * Reduced maintenance costs: PdM helps identify and replace only critical components, eliminating unnecessary maintenance expenses and minimizing spare parts inventory. * Enhanced product quality: By preventing equipment failures, PdM ensures that products meet quality standards and customer specifications. * Improved safety: By identifying potential hazards and addressing them before they cause accidents, PdM contributes to a safer work environment. * Increased productivity: With reduced downtime and improved equipment performance, smart factories can achieve higher productivity levels and maximize output.
How Predictive Maintenance Works
Predictive maintenance in smart factories involves the following steps:
1. Data collection: Sensors installed on equipment collect real-time data on vibration, temperature, pressure, and other parameters. This data is transmitted to a central platform for analysis. 2. Data analysis: Advanced ML algorithms analyze the collected data to identify patterns and anomalies that indicate potential equipment failures. 3. Prediction: Based on the analysis, ML models predict the probability and timing of equipment failures. 4. Action planning: Maintenance teams use the predictions to plan maintenance actions, such as scheduling repairs or replacing components.
Challenges of Implementing Predictive Maintenance
While the benefits of predictive maintenance are substantial, its implementation in smart factories can present certain challenges, such as:
* Data quality: Ensuring the quality and accuracy of the data collected from sensors is crucial for effective PdM. * Algorithm development: Developing and deploying ML algorithms that accurately predict equipment failures requires expertise and ongoing refinement. * Integration with existing systems: Integrating PdM solutions with existing manufacturing and maintenance systems can be complex and time-consuming. * Maintenance expertise: Implementing PdM requires a skilled maintenance team that can interpret the predictions and take appropriate action.
Overcoming Implementation Challenges
Manufacturers can overcome the challenges of implementing predictive maintenance in smart factories by:
* Investing in high-quality sensors: Accurate data collection is essential for effective PdM. * Collaborating with data scientists: Partnering with experts in ML can ensure the development of robust prediction algorithms. * Leveraging vendor expertise: Many PdM solution providers offer integration and implementation support. * Training maintenance teams: Providing comprehensive training empowers maintenance teams to use PdM effectively.
Case Studies
Numerous successful case studies demonstrate the benefits of predictive maintenance in smart factories. For instance:
* Siemens: Siemens implemented PdM in its gas turbine manufacturing facility, reducing unplanned maintenance by 60% and increasing machine availability by 10%. * GE Aviation: GE Aviation deployed a PdM solution for its jet engines, preventing over 100 potential engine failures and saving millions of dollars in maintenance costs. * Schneider Electric: Schneider Electric implemented PdM in its electrical distribution plant, reducing downtime by 30% and improving product quality.
Predictive maintenance is an essential technology for smart factories looking to maximize productivity, minimize downtime, and optimize equipment performance. By leveraging data analytics and ML, manufacturers can proactively address equipment failures, reduce maintenance costs, and enhance product quality. While implementing PdM can present challenges, these can be overcome with strategic planning, collaboration with experts, and ongoing refinement. With the proven benefits of predictive maintenance, manufacturers are well-positioned to embrace this transformative technology and achieve operational excellence in the digital age.
4 out of 5
Language | : | English |
File size | : | 35138 KB |
Text-to-Speech | : | Enabled |
Enhanced typesetting | : | Enabled |
Word Wise | : | Enabled |
Print length | : | 401 pages |
Screen Reader | : | Supported |
Hardcover | : | 430 pages |
Item Weight | : | 1.43 pounds |
Dimensions | : | 6.14 x 0.94 x 9.21 inches |
X-Ray for textbooks | : | Enabled |
Do you want to contribute by writing guest posts on this blog?
Please contact us and send us a resume of previous articles that you have written.
Book
Novel
Page
Chapter
Text
Story
Genre
Reader
Library
Paperback
E-book
Magazine
Newspaper
Paragraph
Sentence
Bookmark
Shelf
Glossary
Bibliography
Foreword
Preface
Synopsis
Annotation
Footnote
Manuscript
Scroll
Codex
Tome
Bestseller
Classics
Library card
Narrative
Biography
Autobiography
Memoir
Reference
Encyclopedia
Audrey Pavia
Bryna Butler
Sudeep Pasricha
Ulrich Daepp
Barry Ahern
B G Pachpatte
K Lang Slattery
Eze Ugbor
Ashkar Ali Kunhikkandy
Tuan Anh Nguyen
Baldev Bhatia
Mark Haber
Athena Simone
Kevin Eason
Barbara Tibbetts
John Boeschen
Julian Woolford
Heidi Nazarudin
Yolanda Ridge
Barb Rosenstock
Light bulbAdvertise smarter! Our strategic ad space ensures maximum exposure. Reserve your spot today!
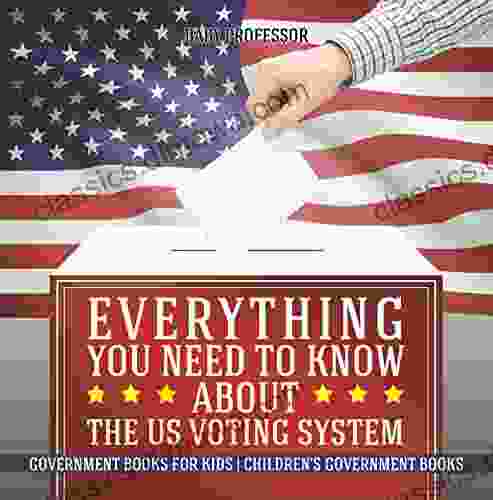

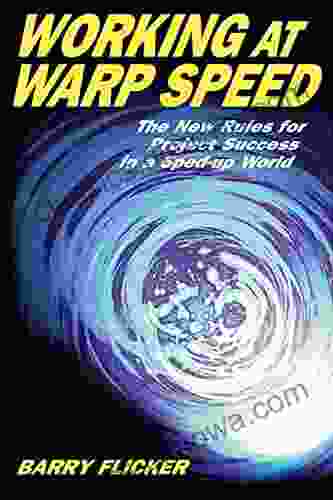

- W. Somerset MaughamFollow ·12.6k
- Vernon BlairFollow ·8.8k
- Jerry HayesFollow ·12k
- Nick TurnerFollow ·19k
- Adrien BlairFollow ·7.9k
- Eric HayesFollow ·15.3k
- Jeff FosterFollow ·11k
- Branden SimmonsFollow ·10k
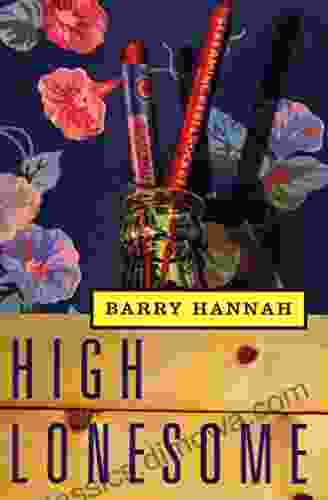

High Lonesome: A Literary Journey into the Heart of the...
<p>Hannah weaves a intricate...
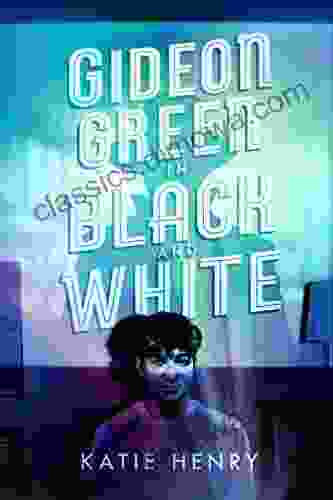

Rediscover Gideon Green's Timeless Adventures in "Gideon...
Embark on an Extraordinary Journey with...
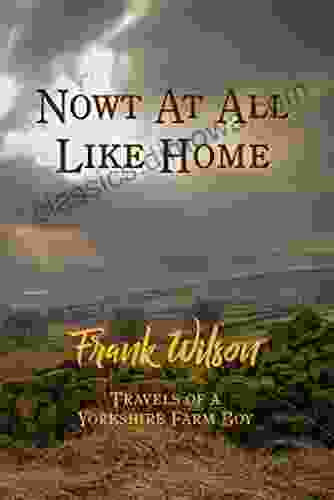

Escape to a Literary Haven: Discover the Enchanting World...
Embark on an Extraordinary Literary...
4 out of 5
Language | : | English |
File size | : | 35138 KB |
Text-to-Speech | : | Enabled |
Enhanced typesetting | : | Enabled |
Word Wise | : | Enabled |
Print length | : | 401 pages |
Screen Reader | : | Supported |
Hardcover | : | 430 pages |
Item Weight | : | 1.43 pounds |
Dimensions | : | 6.14 x 0.94 x 9.21 inches |
X-Ray for textbooks | : | Enabled |